Weights&Biases
Weights & Biases (W&B) is a collaborative platform designed to streamline the machine learning workflow, especially for teams working on deep learning projects.
Tags:AI Developer ToolsAI Developer tools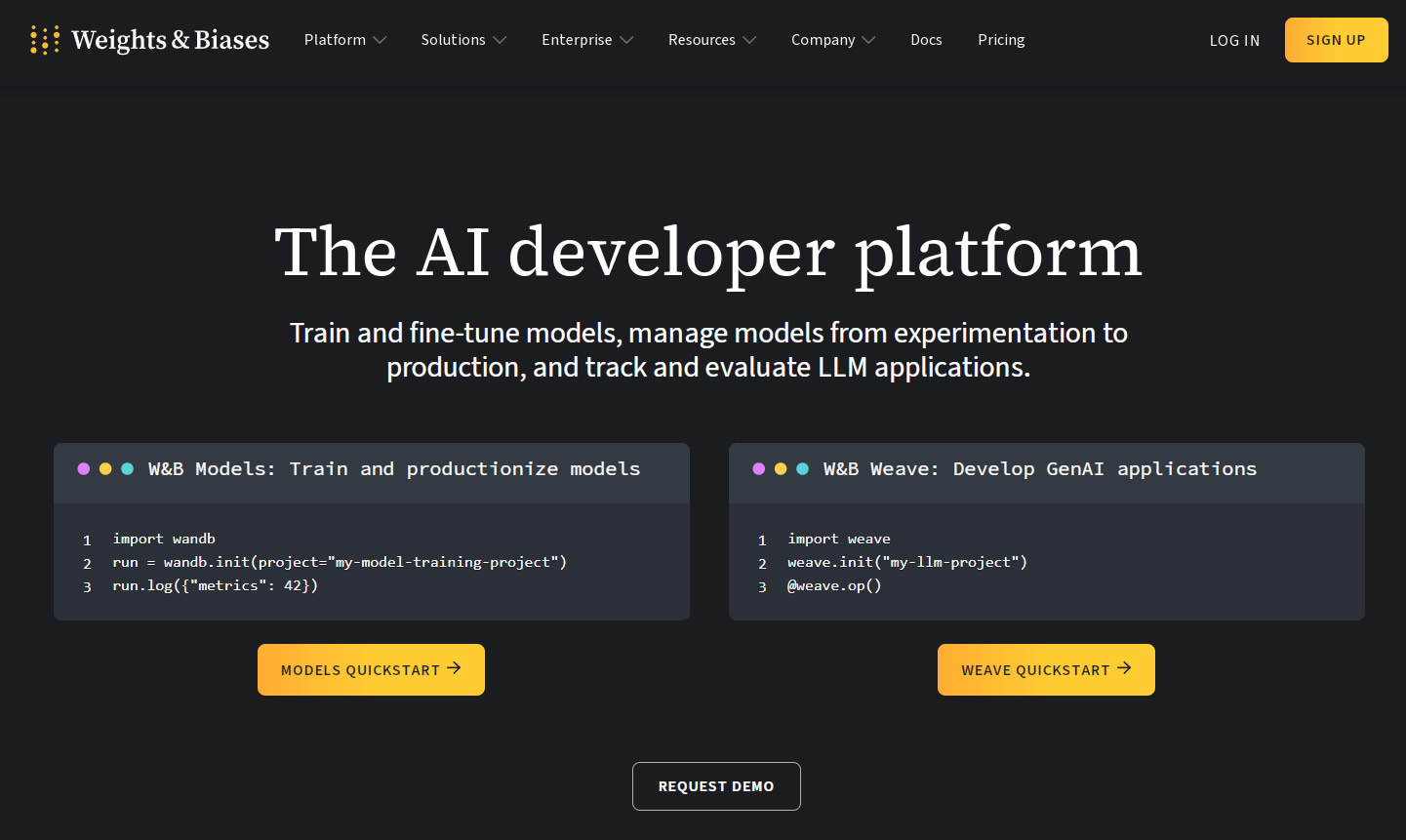
What is Weight&Biases?
Weights & Biases (W&B) is a collaborative platform designed to streamline the machine learning workflow, especially for teams working on deep learning projects. It provides a centralized place to track experiments, visualize models, and share findings, making collaboration more efficient and effective. W&B is particularly useful for researchers, engineers, and data scientists involved in developing and deploying machine learning models.
Key Features:
1.Experiment Tracking: W&B allows users to log and compare experiments easily. It captures metadata about each run, such as hyperparameters, dataset splits, and training/validation loss, making it simpler to reproduce and analyze experiments.
2.Model Visualization: The platform offers tools to visualize model performance over time, helping users understand how changes in hyperparameters or datasets impact model performance. This visualization capability is crucial for diagnosing issues and optimizing model architectures.
3.Collaboration Tools: W&B facilitates collaboration among team members by providing shared dashboards and experiment logs. Teammates can view each other’s progress, discuss experiments, and contribute ideas in real-time.
4.Integration Capabilities: W&B integrates seamlessly with popular machine learning frameworks and libraries, such as TensorFlow, PyTorch, and scikit-learn. This integration ensures that logging and visualization are straightforward, minimizing the overhead associated with adopting new tools.
5.Data Versioning: The platform supports data versioning, allowing users to track changes in datasets over time. This feature is essential for reproducibility and understanding how data transformations affect model outcomes.
Target Audience:
1.Machine Learning Researchers and Engineers: Those involved in developing and testing new machine learning models can greatly benefit from W&B’s experiment tracking and model visualization features. It simplifies the process of iterating on models and sharing findings with colleagues.
2.Data Science Teams: Data science teams working on projects that involve training and evaluating machine learning models can use W&B to streamline their workflows. The platform’s collaboration tools make it easier to coordinate efforts and share insights.
3.Educators and Students: Educators teaching courses on machine learning or data science can use W&B to demonstrate best practices in experiment tracking and model evaluation. Students can learn from real-world examples and gain practical experience with tools that are widely used in the industry.